Scraping utility bills is a commoditized solution at this point. Whether for benchmarking or tenant bill backs, electric, water, gas, and trash bills are being converted from PDFs to a usable format by the tens of thousands.
For the uninitiated, the process is straightforward: a PDF is run through bill scraper technology, from which the cost and consumption is extracted, along with the billing period and the rate charged.
Many, including some who spend hours of their working day correcting issues, don’t appreciate what’s actually happening behind the scenes.
The critical thing to understand is that utility bills are unbelievably variable, and that a lot of the information not needed for benchmarking only adds noise. Even bills issued by the same utility can vary from account to account.
The downstream effect is that bill scrapers systematically fail to handle the nuances between providers, resulting in anomalous data, gaps in coverage, misallocations, and more. From there, portfolios either fail critical audits, hire expensive consultants, or comb through the data in-house to make corrections.
For this reason, some consultants have concluded that software is not the answer — that it’s better for property managers to manually input data.
Fortunately, there’s a better way — if you know what to look for.
What Proactive QA Looks Like
There are three key components to quality assurance that work together to ensure bills are processed effectively, issues are caught and resolved before reaching the user, and that consistency is maintained over time.
- Post Processing
As mentioned, basic utility bill scraping is a commoditized service. Whether done in-house or via a third party, the technology is basically the same.
What happens next — during post-processing — is what really matters. Because it’s never as simple as “give me the cost, consumption, and demand.”
It’s important to understand that 1) utility bills between providers are different (and ever changing), and 2) utility bills between accounts within the same provider have variations.
Let’s look at an example. Here are two bills from the same provider. Try to quickly spot the differences. Now try to think like a computer — one that can follow very precise instructions but can’t reason critically like a human. (Generative AI is helping, but still has a long way to go and can be very confident in wrong answers.)
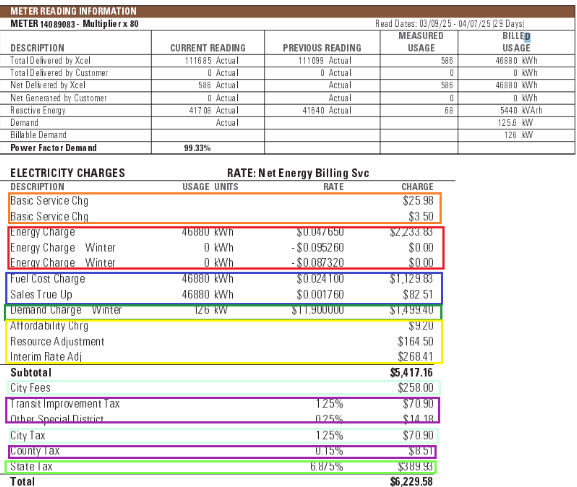
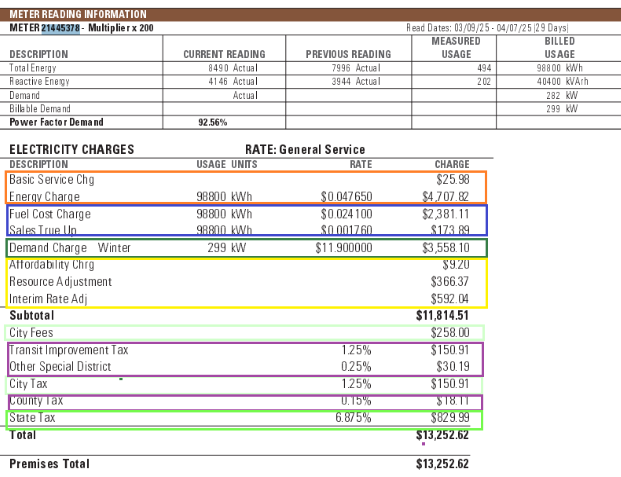
Post processing ensures that all of the many variations are handled.
- Automated Quality Assurance
Effective post processing is a critical first step but will never be perfect.
That’s where automated quality assurance checks come in. Before data is pushed onto a platform (and certainly before it is pushed to Energy Star or ESG reporting frameworks), it should go through a set of rigorous checks.
There are many things that can go wrong, so these checks must cover outlier amounts relative to previous bills, missing data, incorrect units, overlapping billing periods, and much more.
At Enertiv, there are 15 unique checks, a backend validation log, and a severity level for each instance.
- Feedback Loop
It’s one thing to identify (or less ideally, be told by a client) that there’s an error and correct it.
It’s another thing entirely to have a built-in feedback loop that corrects the issue going forward for that specific utility bill format.
This is known as human-in-the-loop — where the process is mostly run by software and AI agents, but humans step in for training and correction.
This approach allows humans in the vendor-client relationship to focus on being trusted advisors, resources, and experts — instead of data-entry machines or punching bags when issues arise.
The Payoff
In the unbundling occurring between E, S and G, firms are realizing just how much weight rests on utility bill data, and how mission critical it is to get that piece right.
For many, utility data is going through audits at the same level of scrutiny as financial data. Investors are expecting this data to be easily provided, even for triple net leased assets where tenants pay the bill.
Likewise, benchmarking standards are proliferating across cities and states/provinces, many of which carry fines for noncompliance.
And while there are certainly sticks, there are also carrots for those with clean, accurate utility data.
These include energy services, such as demand response, where either owner or tenant (depending on who pays the bill) can get 5-15% of their annual utility costs back in cash payments from the utility.
Another example is data-driven energy procurement, where rates can be locked in at the optimal time and/or floated to maximize savings.
Even if these services aren’t used, the truth remains: time spent manually compiling, reviewing, and correcting utility bill data is time not spent on asset optimization, tenant engagement, or decarbonization planning.
In a world increasingly powered by software and AI, no one should have to spend so much of their time on utility bill data management.