How to Identify Tech with Exponential Value Potential
Data is becoming a significant determiner of success in the commercial real estate industry. At the same time, data itself has no inherent value and more data doesn't necessarily mean more value.
In this video, Kevin Shtofman from Deloitte Consulting explains how to identify tech with exponential value potential.
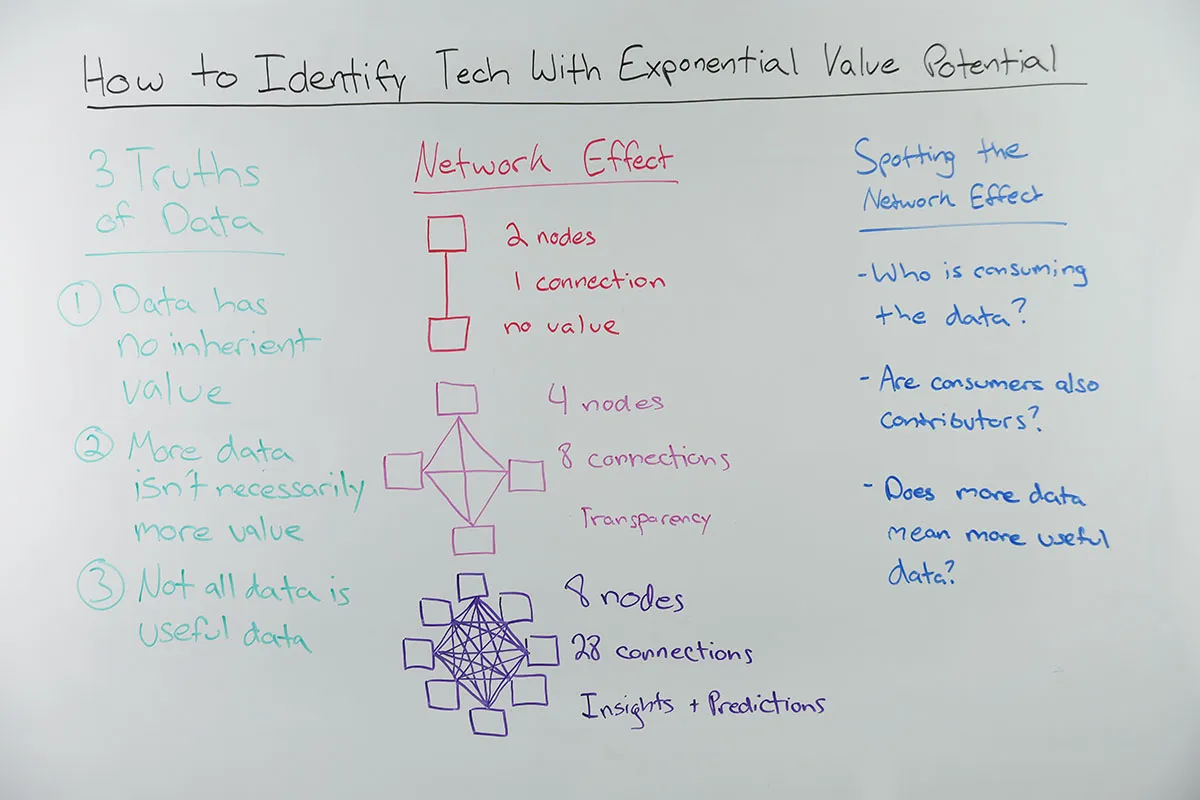
Video Transcript
Hello everyone, I’m Kevin Shtofman. I run a global real estate technology group for Deloitte Consulting, and with that I want to introduce today’s topic – the most common question I get from our global clients.
That is, how do I scale with my data? How do I make my data an exponential value set and not a linear value set? And while most real estate clients are very sophisticated, they come to me with preconceived notions – a lot of myths about their data.
“I gather a lot of data; data is the new oil; it’s going to be so valuable for my company.” Well, let’s use that analogy because everyone seems to use it. Data, aka oil, when you pull it out of the ground, is worthless sludge, it’s a commodity. You have to refine it, transport it to its final destination, and then actually sell it to the consumer or end user. The same is the case with data.
When you gather it by itself, it has no inherent value. On the other hand, when you analyze it, and connect it with other data points, that has a very large potential value. Data can truly be treated as an asset at that point.
The second myth about data is that gathering more of it inherently adds more value. Well that’s not true. If data itself as no value, getting more of it doesn’t increase the value of it as an asset. If I give you your fifth Yelp! review or Amazon rating, that’s very valuable, and has a material change in percentage of rating, but the 100th review or 500th review has very diminishing returns because the scope of what you’re measuring is inherently narrow. The key to get value out of that is to broaden the scope of what you’re measuring.
And that is my third point: not all data is useful data. I can gather a lot of different data points, but if I’m an office developer and I’m measuring user access, weather could be an important component that’s driving who’s coming into the building or out, but knowing that it’s a Sunday, it might not be important.
So, let’s talk about how we scale up the value of data. When most people gather data… I happen to be in the Enertiv offices here, they provide a lot of hardware data value. So, let’s talk about chillers. If I only have two chillers in one building providing data to me, that might be slightly value to me. If I have eight chillers across four different buildings, I can do a lot more comparison. Well, if I have 80 chillers across 100 buildings, I have a massive value potential. I can go from a reactive policy of measuring data and then reacting to it, to actually predicting what my demand might be in certain regions, in certain departments, on certain floors, for certain user groups. It all becomes very valuable.
Having that scale becomes extremely important. So how do I spot that network effect? How do I take advantage of that huge data piece? Well first, I need to know who’s actually consuming my data. If I’m a multifamily developer and I’m trying to dynamically price units on a weekend, I need to know who’s looking at that data. Is it people visiting my site? Is it partners looking to develop elsewhere? Is it competitors that are looking at my pricing data?
Secondly, who’s actually contributing to the system where I’m gathering my data? If CoStar calls me and asks me for a comp, I’m probably not going to be very honest if I’m a developer or an owner-operator myself, I’ll fudge the numbers a bit. If I’m interacting with a software application on my phone, like Waze, I’m going to be very clear about when there’s a traffic jam and because I want to avoid it and help other users. It matters what type of data you are contributing and whether you are a consumer of that data yourself, because then you’re on the same page as everybody else.
Lastly, does more data actually mean more useful data. This goes back to the myth I talked about earlier. More data points can be valuable, but they might be a waste of time, they might be white noise and we don’t want to suffer paralysis by analysis. So, if I’m a corporate owner of real estate, let’s say BestBuy, and I’m looking for a particular location, if I have one comp in one area, that’s fine. But if I’m using a broker that’s working in other regions to compare what BestBuy is paying, that’s more valuable. If they also know who the typical neighbor tenants in an open-air shopping center, that’s even more valuable. And most valuable could be if they know particular local tax incentives or infrastructure improvements that are coming. Broadening that scope of data gives me insights, not just data – that’s how we scale and that’s how we get exponential value for our clients.